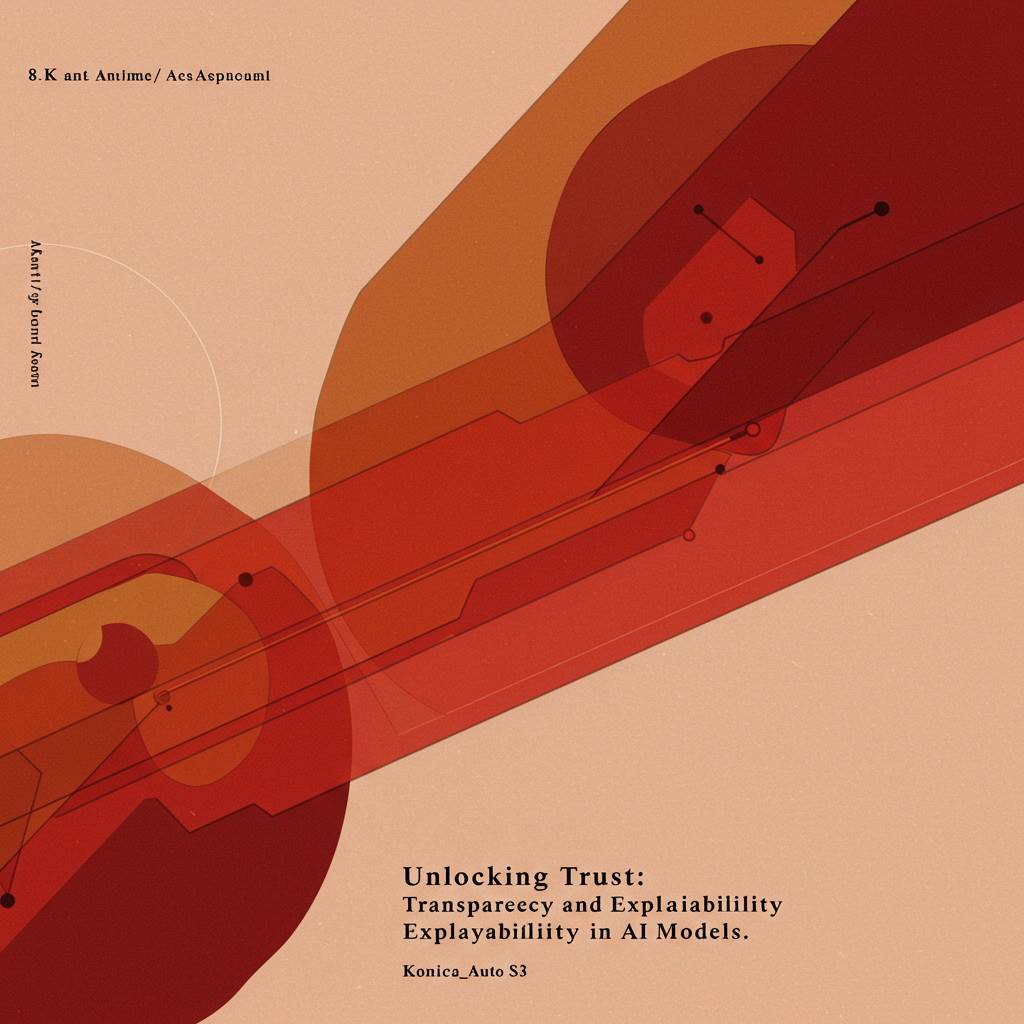
Unlocking Trust: Transparency and Explainability in AI Models
Transparency and explainability are crucial components of AI systems, enabling users to understand how decisions are made and fostering trust in AI-driven outcomes. Here are some key aspects of transparency and explainability in AI models:
-
Building Trust: By providing insights into AI decision-making processes, transparency and explainability help build trust among users and stakeholders. This is particularly important in high-stakes applications, such as healthcare and finance, where AI decisions can have significant impacts12.
-
Addressing Bias and Fairness: Explainable AI (XAI) allows for the identification and correction of biases in AI models. This ensures that AI-driven decisions are fair and equitable, aligning with ethical standards and regulatory requirements34.
-
Regulatory Compliance: Transparency and explainability are essential for compliance with regulations like GDPR, which mandates that individuals have a “right to explanation” for decisions made by AI systems37.
As AI continues to evolve, prioritizing transparency and explainability will remain vital for responsible AI development and deployment.
For more information on transparency and explainability in AI, you can explore the following resources:
-
Ensuring Transparency and Explainability: LinkedIn discusses the importance of these concepts in building trust.
-
AI Transparency and Explainability: TechTarget highlights the need for transparency in AI systems.
-
Explainable AI (XAI): Kolena provides insights into XAI’s role in enhancing fairness and reducing bias.